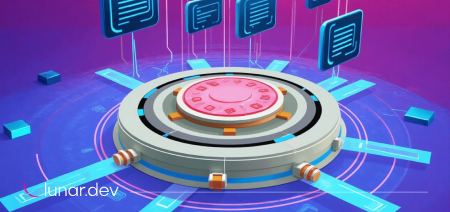
MCP Is Here — This is the Infrastructure It Needs
MCP is evolving into a standard layer in the AI stack. But with that potential comes real infrastructure risk. As the ecosystem grows, decision-makers should keep infrastructure top-of-mind. With the right controls in place, MCP doesn’t just unlock new capabilities—it becomes a trusted foundation for the next generation of AI-powered systems.
Model Context Protocol (MCP) is gaining serious momentum in standardizing how AI agents—like co-pilots and assistants interact with external tools and data sources via a clean client-server abstraction. Rather than building one-off integrations, MCP proposes a universal way to connect models to services—simplifying how AI gets things done across systems.
And it’s already happening - MCP is evolving into a standard layer in the AI stack.
Since its open-source release by Anthropic, MCP has seen fast adoption. Claude 3.5, OpenAI’s desktop app, and Microsoft’s Copilot Studio are all adding MCP support. Even enterprise players are leaning in. Microsoft’s implementation includes enterprise-grade controls like DLP, token security, and network isolation. Meanwhile, startups like RunReveal are using MCP to let customers query observability data with natural language, and tools like mcp.run can auto-generate MCP servers from OpenAPI specs.
Anthropic maintains a growing registry of over 250 MCP servers—from Slack and GitHub to databases—while developers at Replit, Zed, and Codeium are building use cases on top of it.
MCP is evolving into a standard layer in the AI stack. But with that potential comes real infrastructure risk.
The Promise & The Risks
Alongside its promise, MCP raises critical questions around identity, data control, and cost governance. These aren’t new problems—but MCP brings them into sharper focus due to the scale and autonomy that AI agents introduce.
1. Authentication and Identity Ambiguity with MCPs
Today, MCP doesn’t specify how agents authenticate. Without standards for identity delegation or token handling, developers are left to make risky decisions. Should all requests run under one service account? Should AI agents impersonate users? Who's responsible for token lifecycle and permissions?
These are not edge cases—they’re core concerns. Without proper identity boundaries, auditability is lost and the blast radius of compromise expands. As Mark O’Neill noted on LinkedIn, the lack of clear identity semantics creates confusion that will only grow as adoption scales.
2. Data Exposure and Compliance Risks
Pillar Security recently outlined how over-permissive tokens and vague boundaries around agent behavior can result in cross-domain data leaks. Without visibility into what agents are requesting and what data is returned, companies risk violating compliance standards like GDPR, HIPAA, or SOC 2.
Because MCP enables agents to access multiple APIs and services, it’s easy for sensitive data to move between systems—sometimes without users realizing it. A prompt injection or poorly scoped integration could result in an AI assistant to exfiltrate PII, source code, or internal documents.
3. Cost Overruns and Quota Abuse
AI agents don’t inherently understand cost implications. An agent might make thousands of API calls in a loop or request large datasets without considering quotas or billing. When these calls hit rate-limited or metered APIs, things get expensive quickly.
Gartner’s report on third-party API security emphasizes that outbound API usage—especially when driven by SaaS or automated agents—is one of the most under-monitored parts of the enterprise stack. The result? Financial exposure and degraded services.
The Infrastructure MCP Needs
To enable safe, scalable adoption of MCP, organizations need to establish a robust control layer around how AI agents consume external APIs. This is where a modern API consumption gateway comes in.
This gateway acts as the infrastructure layer that governs and secures all outbound API traffic—not just for humans, but for AI agents too. And when AI agents route their actions through this layer, it effectively becomes an AI gateway: shaping behavior, enforcing policies, and maintaining observability.
Key capabilities of such an infrastructure include:
- Endpoint-level filters: Control which specific endpoints or services can be accessed, under what conditions.
- Rate limiting and quota enforcement: Prevent runaway agents from exhausting API quotas or causing bill spikes.
- Token lifecycle and injection: Manage secure token rotation, limit scope, and inject credentials at the proxy level—so agents never touch sensitive secrets.
- Data loss prevention (DLP): Scan outbound payloads for sensitive data patterns before they leave your boundary.
- TLS/mTLS encryption: Ensure all communication is secure and authenticated.
- Audit logging and anomaly detection: Track usage patterns, detect anomalies, and provide visibility into agent-initiated requests.
Looking Ahead
While it’s tempting to focus on the possibilities—speed, automation, reach—it’s equally important to plan for safe, cost-efficient, and compliant adoption. Security and control won’t slow MCP down—they’ll help it scale responsibly.
Lunar.dev offers a comprehensive platform for managing this stack. Our infrastructure routes AI-driven API traffic through a unified control plane—giving teams the tools to set active policies and manage budgets.
MCP is evolving into a standard layer in the AI stack. But with that potential comes real infrastructure risk.
As the ecosystem grows, decision-makers should keep infrastructure top-of-mind. With the right controls in place, MCP doesn’t just unlock new capabilities—it becomes a trusted foundation for the next generation of AI-powered systems.
Ready to Start your journey?
Manage a single service and unlock API management at scale